Title: Processing Data in the era of Artificial Intelligence
Artificial Intelligence (AI) has revolutionized the way we process data, enabling us to extract valuable insights from vast amounts of information. In this article, we will explore the various techniques and tools used for data processing in the context of AI, as well as their applications in academic research and writing.
Introduction
Data is the lifeblood of modern society, and its analysis is a critical component of many fields, including artificial intelligence. As the amount of data generated continues to grow at an exponential rate, the ability to efficiently process and analyze this data has become increasingly important. Fortunately, advances in AI technology have made it possible to automate many aspects of data processing, significantly improving our ability to extract meaningful insights from complex datasets.
Data preprocessing
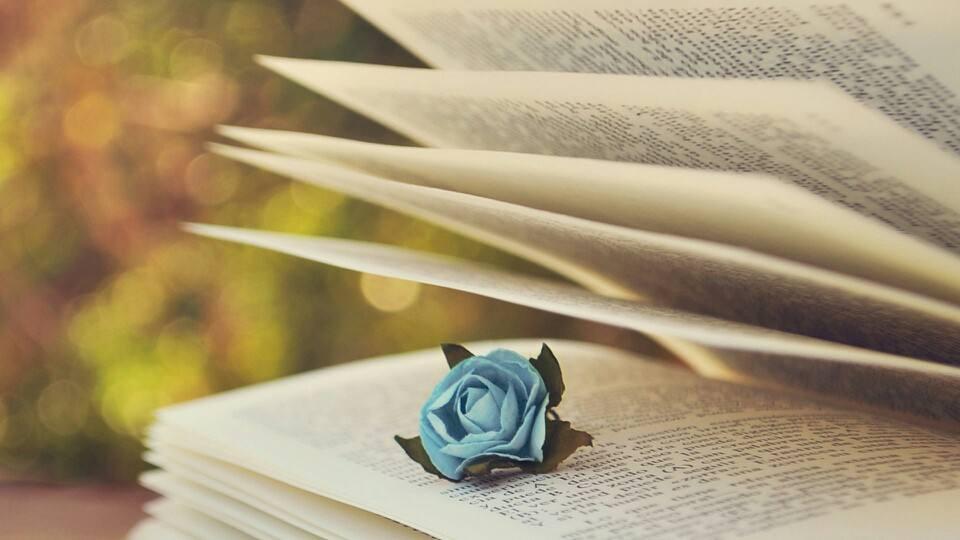
Before any analysis can be performed on the data, it must first be cleaned and prepared for analysis. This involves tasks such as removing missing values, handling outliers, and normalizing the data. There are several techniques for preprocessed data, such as normalization, scaling, and feature selection. These techniques help ensure that the data is suitable for further analysis, which can lead to more accurate and reliable results.
Feature engineering
Feature engineering is the process of selecting or creating relevant features from raw data that can be used to train machine learning models. In some cases, raw data may not contain all the necessary features for analysis, so feature engineering becomes essential. Feature engineering can involve a wide range of techniques, including principal component analysis (PCA), linear regression, logistic regression, and decision trees. The goal of feature engineering is to identify the most important features that can accurately predict outcomes or classify data.
Machine learning algorithms
Machine learning algorithms are designed to learn patterns and relationships from data. Once the features have been engineered, these algorithms can be trained on the preprocessed data to make predictions or classifications. Some common machine learning algorithms include linear regression, logistic regression, decision trees, random forests, support vector machines (SVMs), and neural networks. Each algorithm has its strengths and weaknesses, and choosing the right algorithm depends on the nature of the problem and the available data.
Applications in academic research and writing
The field of artificial intelligence has numerous applications beyond just processing data. One area where AI is making a significant impact is in academic research. Researchers use AI algorithms to analyze large-scale datasets and discover new patterns and relationships that might not be apparent through manual analysis. This can lead to breakthroughs in fields such as medicine, physics, and chemistry. In addition, AI can also assist in the writing process by providing suggestions for word choice, sentence structure, and other aspects of text generation. This technology allows researchers and writers to spend more time focusing on creative aspects of their work rather than spending hours manually analyzing data.
Conclusion
Processing data using artificial intelligence has become an essential part of modern research and writing. By automating many aspects of data processing, AI enables researchers to focus on higher-level tasks that require human ingenuity. With the continued development of AI technology, we can expect even more powerful tools for analyzing and interpreting data in the future.