Data Processing: The Abbreviation in English for Handling Data
Introduction:
Data processing plays a pivotal role in today’s era of artificial intelligence where the amount of information generated is colossal. This article AIms to shed light on the English abbreviation for data processing, focusing on its significance in the field of AI and its relevance to scholarly writing. Furthermore, we will explore the connection between data processing and plagiarism detection, as well as methods to reduce plagiarism in academic papers when using data processing techniques.
Body:
1. The English Abbreviation for Data Processing:
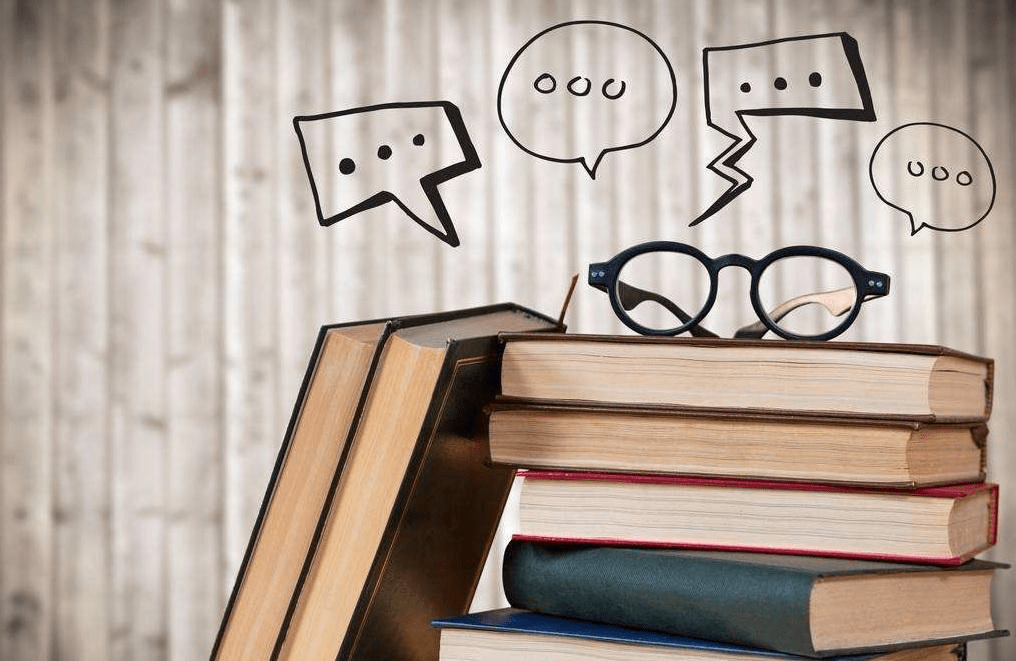
In the realm of artificial intelligence and computing, the abbreviation commonly used for data processing is DP. This term refers to the transformation of raw data into useful and meaningful information through various techniques and algorithms. DP encompasses a wide range of Operations, including data cleaning, integration, analysis, and visualization.
2. Data Processing in Artificial Intelligence:
Data processing serves as the foundation for the development and implementation of AI models. In this context, AI algorithms heavily rely on large datasets to perform tasks such as machine learning, natural language processing, and computer vision. Through advanced data processing techniques, AI systems can uncover patterns, make predictions, and deliver accurate results.
3. Data Processing and Scholarly Writing:
In academic research, data processing is essential for conducting experiments, analyzing results, and presenting findings. Researchers utilize statistical software and programming languages to process collected data, ensuring its reliability and validity. Data processing enables scholars to transform raw data into comprehensive and structured formats, facilitating the interpretation of outcomes and supporting the overall argument in scientific papers.
4. Data Processing and Plagiarism Detection:
Through data processing techniques, plagiarism detection in academic writing has become more refined and efficient. Plagiarism detection tools utilize algorithms to compare submitted papers against a vast database of existing literature. By processing data from both the submitted paper and external sources, these tools can identify similarities and instances of plagiarism, thereby ensuring the originality and integrity of scholarly work.
5. Reducing Plagiarism in Academic Papers:
While data processing aids in plagiarism detection, it is equally important to employ strategies to minimize the risk of unintentional plagiarism. Firstly, it is crucial to cite all sources accurately and provide proper attribution. Secondly, paraphrASIng should be used to express ideas in one’s own words while still acknowledging the original source. Finally, using plagiarism detection software during the writing process can help identify potential issues and offer suggestions for rephrasing or reorGANizing content.
Conclusion:
In the realm of artificial intelligence, data processing serves as the backbone of various AI applications. Its significance in academic writing cannot be overlooked either, as it enables researchers to derive insights from data and present their findings effectively. Moreover, data processing has revolutionized plagiarism detection, allowing for enhanced originality and research integrity. By understanding the abbreviation for data processing in English and its broader implications, scholars and AI enthusiasts can harness its power to advance scientific discoveries while maintaining ethical writing practices.